Unlocking the Power of Image Classification Labeling Tool
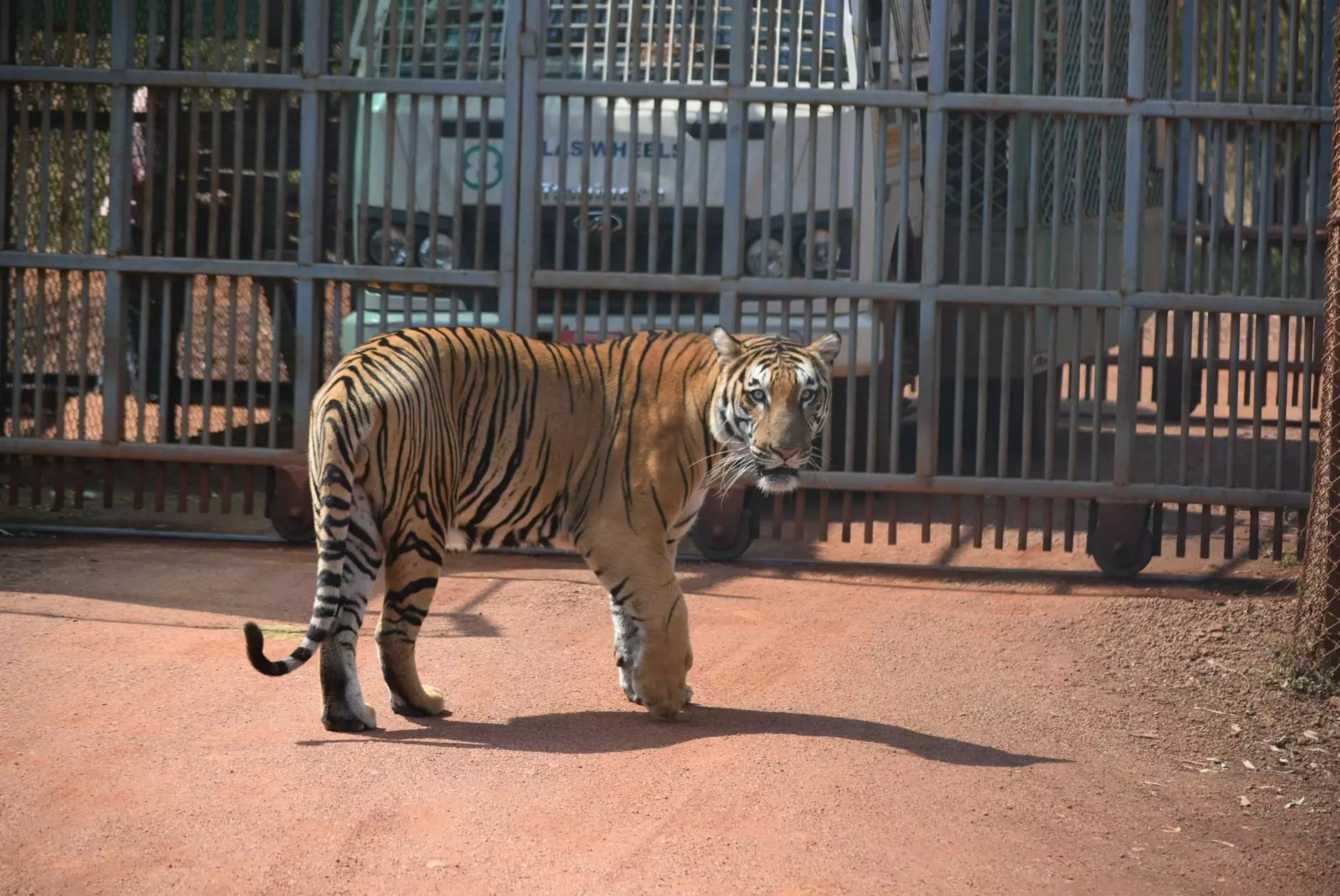
In the fast-evolving world of technology, businesses are increasingly relying on data annotation to train their machine learning models effectively. One crucial part of this ecosystem is the image classification labeling tool, a pivotal instrument in enhancing the quality of data used for training AI systems. In this article, we will explore the significance of such tools, the various aspects of data annotation, and how platforms like keylabs.ai are revolutionizing this landscape.
Understanding Image Classification Labeling Tools
At its core, an image classification labeling tool is designed to assist data annotators in tagging images with the appropriate labels. This process is essential in creating labeled datasets that machine learning algorithms use to learn and make predictions. Without these labeled datasets, the potential of machine learning algorithms would remain unrealized.
What is Image Classification?
Image classification involves categorizing images into predefined classes based on their visual content. For instance, an image classification model could differentiate between various types of animals, objects, or even facial expressions. The accuracy and efficiency of these classifications heavily depend on how well the images are labeled during the training phase.
The Importance of Data Annotation
Every machine learning model thrives on data, and the quality of that data is paramount. Data annotation serves as the foundation for training these models. Here are several reasons why data annotation, especially through image classification labeling tools, is crucial:
- Enhanced Model Accuracy: Good data quality leads to improved model predictions and accuracy.
- Efficient Learning: Well-annotated datasets enable faster learning for algorithms, reducing training time significantly.
- Scalability: As businesses grow, so does the volume of data. Effective data annotation tools can manage large-scale projects seamlessly.
- Cost-Effectiveness: Automating parts of the annotation process can save both time and resources, making it a financially wise decision.
Features of an Effective Image Classification Labeling Tool
When looking for an image classification labeling tool, it’s vital to consider specific features that enhance usability and productivity. Here are some critical features to look for:
1. User-Friendly Interface
A simple and intuitive interface ensures that annotators can work swiftly without extensive training. The easier it is to navigate, the less time is spent on onboarding.
2. Multi-format Support
As modern businesses utilize various types of images, it’s essential that an image classification labeling tool supports multiple file formats like JPEG, PNG, and TIFF.
3. Batch Processing Capabilities
Batch processing allows annotators to label multiple images simultaneously, increasing productivity and efficiency.
4. Quality Assurance Features
In-built quality assurance mechanisms, such as review features and consensus checks, help maintain high-quality annotations.
5. Integration with ML Frameworks
Compatibility with popular machine learning frameworks and libraries enhances the workflow by allowing seamless transitions from annotation to model training.
The Data Annotation Process
Understanding the data annotation process is crucial to grasp how an image classification labeling tool fits into the bigger picture. Here’s a step-by-step overview:
Step 1: Dataset Collection
The first stage involves gathering images that will be used for the training set. This can include anything from photographs and drawings to diagrams and screenshots.
Step 2: Image Preparation
After collection, the images are prepared for annotation. This might include resizing, normalizing, or modifying images to ensure consistency across the dataset.
Step 3: Annotation
This is where the actual labeling happens. Annotators use the image classification labeling tool to add labels that correlate with particular elements within the images.
Step 4: Quality Control
Once images are labeled, they undergo a quality control process where accuracy is checked, ensuring that the labeling meets the set standards.
Step 5: Model Training
Finally, the labeled data is used to train machine learning models. The better the labeling, the more accurate the model will be in its predictions.
Challenges in Image Annotation
While image classification labeling tools are incredibly useful, the data annotation process does come with its challenges, including:
- Subjectivity: Labeling can often be subjective, leading to inconsistencies in how images are tagged.
- Time-Consuming: Depending on the scale, annotating data can take significant time and effort.
- Cost Factors: Hiring expert annotators or using premium tools can increase operational costs.
Choosing the Right Data Annotation Platform
When considering a data annotation platform, particularly for an image classification labeling tool, evaluating a range of options based on specific needs is paramount. Here are some tips when choosing the right platform:
- Assess Flexibility: Ensure the platform can adapt to your specific project needs, whether it’s the type of images or the kind of classification required.
- Evaluate Scalability: Look for platforms that can grow alongside your business, accommodating larger datasets as they come in.
- Check Reviews: Investigate user experiences and satisfaction levels with the platform to gauge reliability and efficiency.
- Price Structure: Understand the pricing model to ensure it fits your budget while still providing value for features offered.
Future Trends in Image Annotation
As technology continues to advance, so do the methodologies and tools associated with data annotation. Some anticipated trends in the field include:
1. AI-assisted Annotation
The incorporation of AI in aiding the annotation process speeds up labeling. AI can recognize patterns and suggest labels, reducing the annotator's workload.
2. Real-Time Collaboration
Future tools are likely to include real-time collaboration features allowing teams to work simultaneously and share insights instantly.
3. Enhanced Automation
Automation is expected to streamline many tedious tasks, allowing human annotators to focus on more complex elements of the data.
Conclusion: The Gateway to Efficient Machine Learning
In the realm of machine learning, the significance of an image classification labeling tool cannot be overstated. These tools not only facilitate the accurate labeling of data but also set the foundation for developing robust and efficient machine learning models. As platforms like keylabs.ai continue to innovate, businesses can harness the full potential of their data annotation efforts, leading to superior outcomes in training AI systems.
By embracing sophisticated data annotation strategies and leveraging state-of-the-art image classification labeling tools, companies position themselves for success in the competitive landscape of artificial intelligence. The future is bright for businesses that prioritize data quality, and it all starts with effective labeling.